Student Programs
Start Your Career with Us
The Defense Science and Technology Internship (DSTI) prepares graduate and undergraduate students for experimental, theoretical, and computational research in many disciplines, such as:
- Astrophysics
- Hydrodynamics
- Turbulence
- Plasma physics
- Inertial confinement physics
- Radiation/particle transport
We have multiple opportunities for student interns to join a dynamic research environment. Participating students are assigned a research mentor and a project that coincides with the student’s research interests. The interns have the opportunity to interact with staff scientists, give oral presentations, and learn about other interesting research at LLNL. Internships are available for three-month time frames during the summer.
Interns use state-of-the-art simulation codes and have access to world-class facilities including lasers, pulse power machines, and massively parallel supercomputers. The DSTI program includes a summer lecture series and other technical and social activities.
Sample Student Projects
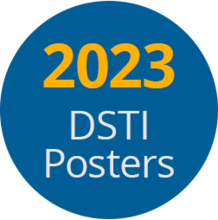
- Hypersonic Shock-Droplet Interactions
- The Four Stages of a Jet Induced by the Collapse of a Gas Bubble
- Gamma-Ray Cascade and Detector Characterization for Indirect Neutron-Capture Measurements
- Using Tensor Trains to Solve High-Dimensional PDE
- Umpire: Performing Experiments to Determine Consistency of Coalescing Heuristics Across Different Memory Pools
Additional Student Opportunities at LLNL
Many summer job openings are posted through one of the Laboratory's scientific discipline organizations such as Computation, Engineering, and Physical and Life Sciences, with assignments directly contributing to the fundamental research needed for our national security mission.
Find additional opportunities and information at: